
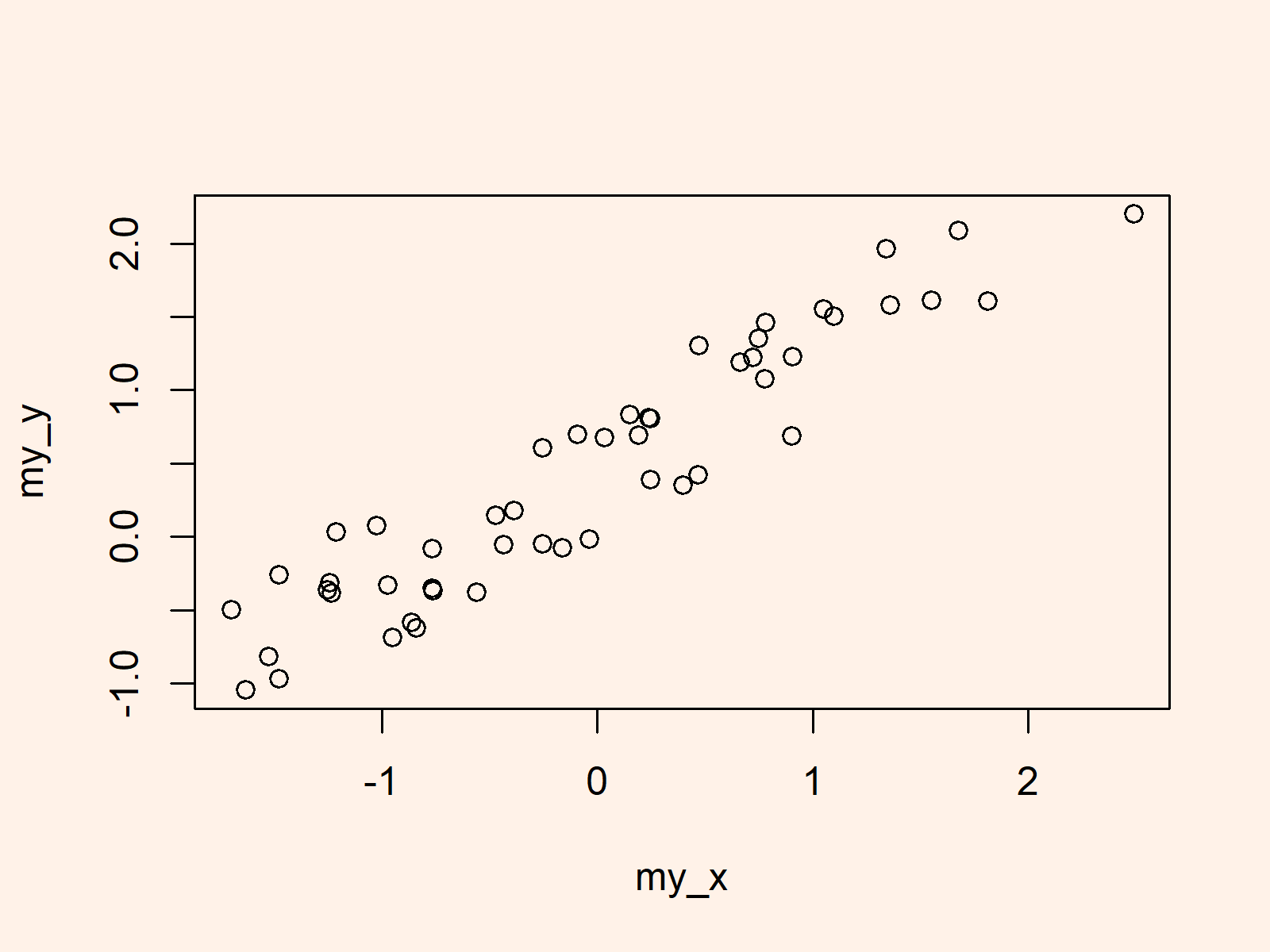
These are incidentally also the first two of the lm(.)-function. The two arguments you will need most often for regression analysis are the formula and the data arguments. The second most important component for computing basic regression in R is the actual function you need for it: lm(.), which stands for “linear model”. The tilde can be interpreted as “regressed on” or “predicted by”. The basic way of writing formulas in R is dependent ~ independent. The initial scatterplot already suggests some support for the assumption and – more importantly – the code for it already contains the most important part of the regression syntax.
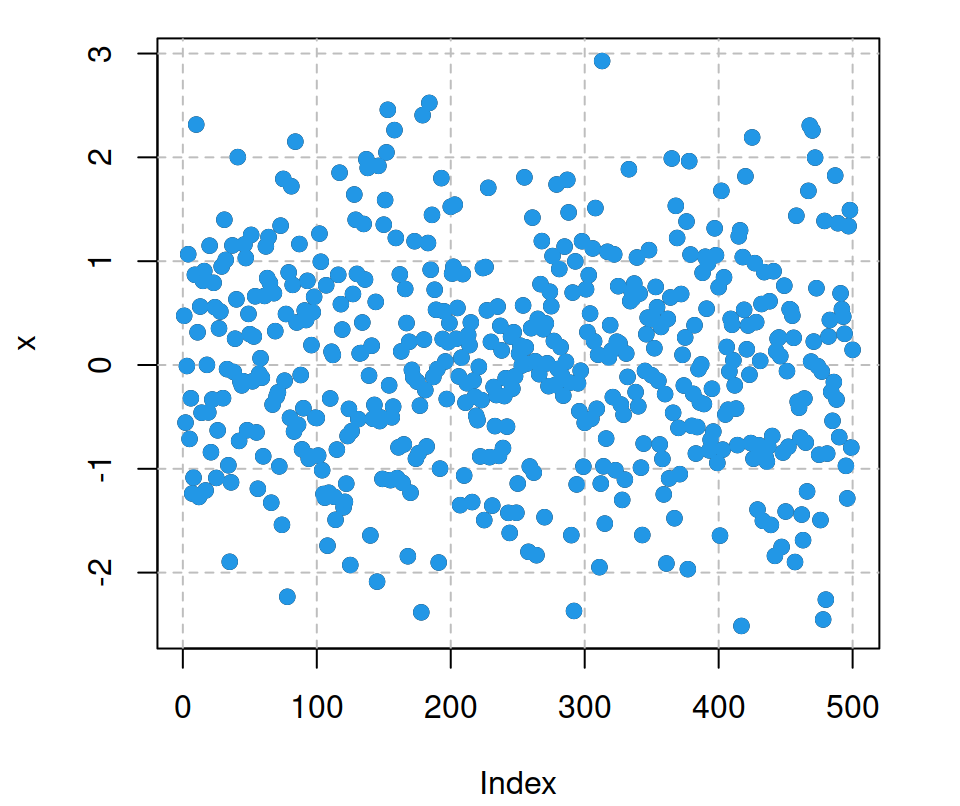
The outcome I’ll be taking a look at here is the Fertility indicator as predictable by the education beyond primary school – my basic assumption being that higher education will be predictive of lower fertility rates (if the 1880s were anything like today).įirst, lets take a look at a simple scatterplot: plot(swiss$Fertility~swiss$Education) To load it into your workspace simply use data(swiss)Īs the helpfile for this dataset will also tell you, its Swiss fertility data from 1888 and all variables are in some sort of percentages. I’ll use the swiss dataset which is part of the datasets-Package that comes pre-packaged in every R installation. Let’s take a look at an example of a simple linear regression. Another great thing is that it is easy to do in R and that there are a lot – a lot – of helper functions for it. The reason for this is that the framework under which regression can be put is both simple and flexible. Multilevel analysis and structural equation modeling are perhaps the most widespread and most obvious extensions of regression analysis that are applied in a large chunk of current psychological and educational research. It forms the basis of many of the fancy statistical methods currently en vogue in the social sciences. Regression is one of the – maybe even the single most important fundamental tool for statistical analysis in quite a large number of research areas.
